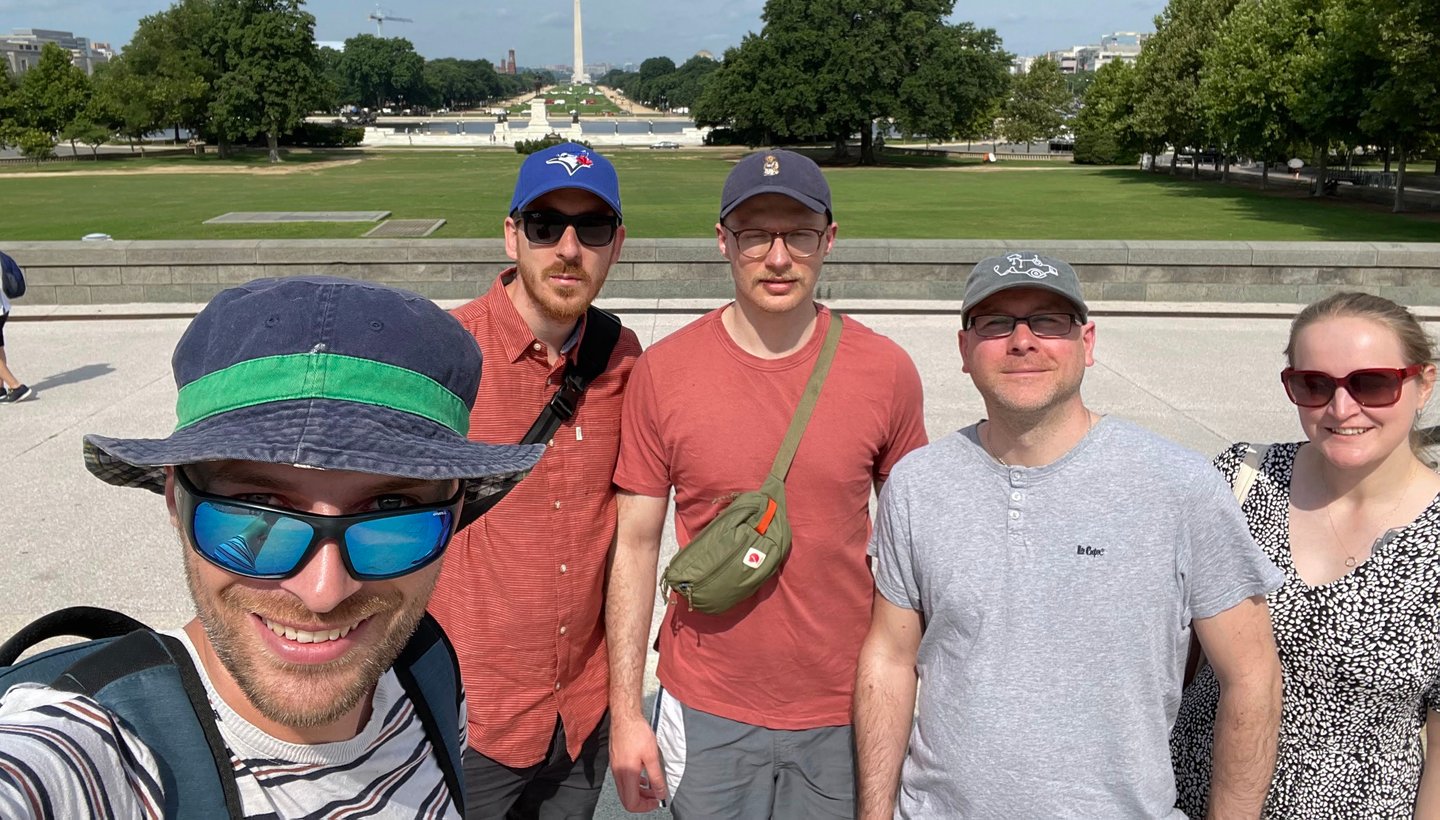
The 39th International Conference on Machine Learning
ICML (aka the International Conference on Machine Learning) is one of the largest annual gatherings of machine learning practitioners and features cutting-edge research on all aspects of machine learning including AI, statistics, and data science. Talks cover research from areas including machine vision, computational biology, interpretable ML, topology, generative models, and from highly theoretical work to novel applications and everything in between.
A team of data scientists from the National Innovation Centre for Data, including myself (Dr Hollie Johnson), attended the thirty-ninth ICML in July this year, held in Baltimore, Maryland. With our diverse backgrounds in statistics, machine learning, computer vision, visualisation and environmental modelling, we each went to ICML hoping to discover new research to guide the development of our own individual skills and to pass on to the organisations we work with.
The main conference days included longer twenty-minute talks and short five-minute pitches within themed sessions, as well as keynote talks. On the final two days of the conference, there was also a selection of full-day workshops on a variety of topics. These tended to include longer talks and posters on a theme and provided a great opportunity to really deep-dive into a topic. We also attended the expo, where global companies such as Google, Meta and AstraZeneca were talking about some of their research.
In two of the keynote talks, Regina Barzilay and Aviv Regev, both from the Massachusetts Institute of Technology (MIT), discussed ways in which machine learning could help solve highly complex problems in the life sciences sector – particularly those relating to drug discovery and validation. It is evident that there is a rapidly growing body of research on ML for life sciences, with many of the research questions being driven by real clinical needs, and drawing on research on graph neural networks in particular.
A new type of hybrid conference
In light of ongoing concerns about Covid-19, calls to make conferences more accessible to wider audiences, and the argument that we should be trying to reduce international travel, ICML should be commended for their efforts to hybridise the conference this year. That said, whilst well thought-out for remote attendees, in reality the hybrid approach resulted in a strange conference experience for those of us attending in person. For example, all speakers were required to pre-record their talks for remote audiences and, unfortunately, many speakers chose to play their video rather than speak live despite being physically in attendance at the conference. We even observed a few using automated text-to-speech! A great demonstration of the technology, but not a very engaging audience experience.
A small number of workshops took a different approach, insisting that all speakers presented in real time (whether in-person or via Zoom), with talks also recorded for remote audiences. The difference this made to the level of engagement in the room was significant and the experience was overwhelmingly more enjoyable. However with this approach, the potential for technical issues is of course increased, so there is clearly some way to go in finding the right balance. We are interested to see how conferences evolve in the coming years to address the ever-changing needs of attendees.
Thoughts from the NICD team
Other members of the team have given their personal highlights below:
Hollie: "The workshop on topology, algebra and geometry in machine learning was a great session. All the talks were live and everyone in the workshop had interesting research and ideas to share. Some of the research was related to my PhD in statistical topology, and it was nice to step back into the topology world for a day and see how those ideas could be used in machine learning."
Mac: “I was delighted to observe a growing research interest in Interpretability and Uncertainty Quantification of machine learning models. These two research topics are imperative for reliable data-driven decision-making.”
Matt: "My highlight of ICML was the workshop on Distribution-Free Uncertainty Quantification. Methods in this field of study can generate confidence intervals for ML model predictions. Unlike some previous methods, these do not depend on assumptions about the ML model or the data distribution. I think applications with high trust requirements (e.g., healthcare) are going to benefit hugely."
Fergus: "I particularly enjoyed a workshop on Data-Centric AI, which focuses on improving data used to train machine learning models. Andrew Ng's keynote about the Data-Centric AI Competition told an engaging story about some innovative ideas that the teams developed and why we need a better way of sharing datasets."
John: "At this year’s ICML I was delighted to see how many sessions focussed on the very real problems of interpreting and validating ML models. I was particularly impressed with GAM Changer - a tool which allows domain experts to tweak the learned functions in additive models."
On our day off, we all visited Washington, D.C., for some well-earned touristing!
Dr Hollie Johnson is a Data Scientist at the National Innovation Centre for Data whose technical background is rooted primarily in statistics and machine learning. Her PhD focussed on topological event history analysis for global climate data and she has previously worked in software development roles.