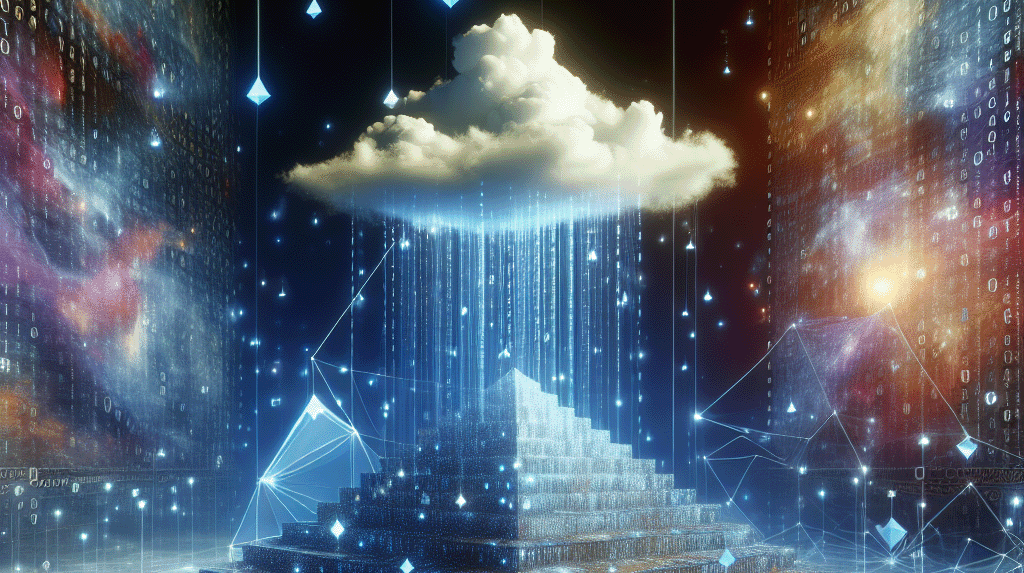
Introducing the latest blog, how not to start a data science project...
Written by NICD Data Scientists Louise Braithwaite MSc and Dr Matt Edwards
Before diving headfirst into analytics, algorithms and AI, let's hit the breaks and take a moment to discuss the less riveting side of starting a data science project. This blog post will shine a light on the common missteps that can throw your data science journey off course. Buckle up and prepare to navigate the pitfalls of, "How not to start a data science project..." together.
So, what is a data science project?
Collaboration across departments is common in data science projects and can involve experts from various domains. Hence, it is wise to establish clear definitions to minimise confusion. So what exactly constitutes a data science project? Let's delve into that.
Definition: A data science project aims to develop a new data-driven product, service or process - or improve an existing one.
Let’s break this down.
First of all, products, services or processes are data-driven when they have components that require data. For example, Netflix streaming service can be considered a data-driven service because it utilises a recommendation system to present users with content they are most likely to watch and enjoy. The recommendation system requires data to make these predictions. Data driven products, services and processes should add value to an organisation. Netflix's recommendation system provides value to the business by increasing user engagement and customer satisfaction, resulting in less customer churn (the number of customers cancelling their subscriptions).
In contrast, a data science project is *not* a project that uses data to explore opportunities to develop new products, services or processes, or improve existing ones. We define these as data exploration projects. Unlike data science projects data exploration projects cannot have well-defined goals based on business value because the value is unknown.
So, data science projects should be aligned to organisational value?
Yes! Data science projects that are aligned to organisational value are more meaningful and impactful because they are more likely to result in projects that:
- address the most pressing business needs
- make the best use of resources
- encourage buy-in and support from stakeholders
- have a measurable benefit
- align with the organisations strategic direction.
Despite the strong motivation to get this right it can be tricky to get projects moving in the right direction. In the following sections we will identify the secret to starting a data science project and some of the common problems organisations face with this task.
The secret to starting a data science project
The secret to starting a data science project is to scope it properly. This involves identifying the right goal and then aligning the objectives, deliverables, and resources to this goal.
The Right Goal
The right goal will have consensus across all relevant stakeholders, be well-defined and describe the value to be delivered to the organisation. Organisational value can usually be categorised in terms of new or improved products and services that can generate revenue or a new or improved process that can reduce costs. This is an important part of a goal being well-defined. All projects are funded to deliver value to the organisation. If this value is not made explicit there is space for ambiguity.
Goal Alignment
Objectives, deliverables, and resources should all be aligned with the project goal. This alignment process encourages efficiency. Only resources that are required to support the delivery are used, only deliverables that are required to support the objectives are developed and only objectives that support the goal are included.
Identify Success Criteria
Measurable objectives provide success criteria. These success criteria describe exactly what it means for part of the project to succeed. Without a definition of success (and failure) it is impossible to pivot when things do not go to plan. Stakeholders can be blind to how far a project is from success and continue to fund it despite failure being a likely result.
Focus on What is Achievable
At the project scoping phases it is important to be bounded by what is realistically achievable and ensuring you can deliver a minimum viable product. Only develop and deploy what is necessary to achieve your objectives. There is always time after a project success to extend certain parts. Any extensions should be approved by the relevant stakeholders as new objectives in a separately scoped data science project.
Interesting Deliverables
Data science teams are often very enthusiastic and keen to explore new state-of-the-art tools and techniques. Without well-defined deliverables, the scope of the project can begin to creep and what is delivered can become more interesting to the data scientists than supportive of the project goals. Ensuring the deliverables and their requirements are well-defined and in alignment with the project goals goes a long way to ensure this does not happen.
Selecting the Right Data
Organisations often have a lot of data. More data than is possible to explore and use in a single data science project. By determining what data is required for the deliverable all irrelevant data can be ignored. Time and effort can be saved in the cleaning and wrangling of this data. Also identifying what data is required but not available or of sufficient quality allows more time and effort to be directed towards data acquisition. This could be collecting or purchasing new data.
Common Problems
Ensuring Consensus
Data science projects often present opportunities to collaborate across departments. However, stakeholders from different departments often have very different perspectives and motivations. This can make it challenging to gain consensus about the goal. Without consensus projects can stall.
Articulating a Clear and Well-defined Goal
If the project goal is not well-defined stakeholders with different perspectives and motivation might interpret the goal differently. This can result in a false perception that consensus has been reached. This can be costly if this is not discovered until late into the project after significant investment has been made. That is why it is important to make sure the goal is unambiguous and communicated affectively to all stakeholders before continuing.
Too Ambitious
Ambitious projects need to be broken down into achievable stages. When projects are too ambitious it becomes much harder to identify realistic objectives and clear deliverables.
Technical Skills Dictating Deliverables
Data science projects require specific skills and expertise to develop the deliverable. The ability to scope a data science project that aligns skills to the deliverable is important. If this is not done a data science team might be tempted to deliver what their skills allow rather than what the objectives dictate. Remember if the deliverable is not in alignment with the objectives, which are in alignment with the goal, there is no reason this deliverable will ultimately deliver value to the organisation at the end of the project.
Confusing a Data Exploration Project with a Data Science Project
Sometimes data science teams are tasked to assist with innovation projects, which aim to use data to explore new opportunities. Data exploration projects cannot have well-defined goals based on business value because the value is unknown. For these types of projects a different framework is needed to support a data science team to identify areas to explore and prioritise where to start.
If you would like help scoping a data science project please reach out to the team to discuss our one-day intensive data science project scoping workshop. Contact nicd@newcastle.ac.uk.
Read more technical blogs from our expert Data Scientists.